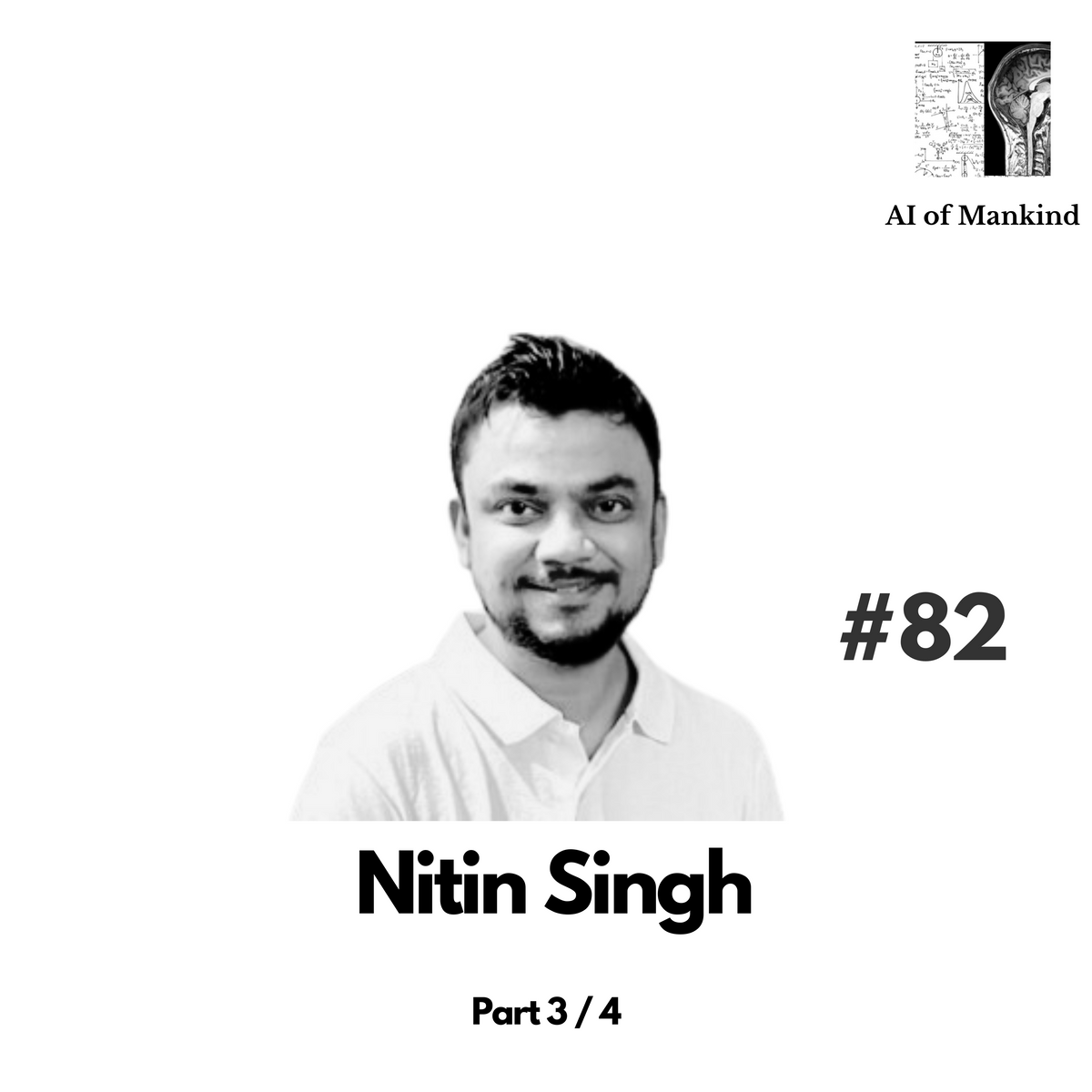
Podcast with Nitin Singh Part 3
Summary: In this podcast, Andrew Liewinterviews Nitin Singh, an AI expert, discussing the future of AI and
technology. They explore various topics, such as the significance of image
analytics and the challenges in NLP and speech recognition. They delve into the
impact of chat GPT and the evolving research landscape. Nitin also emphasizes
the importance of AI governance to address potential risks and deepfake issues.
In this insightful podcast, NitinSingh sheds light on the fast-evolving world of AI and technology. He discusses
the growing importance of image analytics and the challenges in NLP and speech
recognition. Nitin shares valuable insights into the impact of chat GPT and the
changing research landscape. He highlights the need for AI governance to manage
potential risks and issues related to deepfakes. Throughout the conversation,
Nitin emphasizes the value of staying updated and continually upskilling to
harness the true potential of AI.
[00:00:00] Andrew Liew: And when I say that Molody has aunique group of leaders and the speed of execution that draws you to say, Hey,
I'm just going to try this startup rafting, rough waters, instead of climbing a
very big mountain that I'm already there. Is that how, is that the explanation
to how you transit from a big company to a small nimble
[00:00:20] Nitin Singh: fast company?
[00:00:21] Nitin Singh: Yeah, you learn a lot, man, you,yeah, that that's, basically the difference and you have to be very on the feet
you have to be very quick as well. You cannot spend a whole week coming up with
a report or analysis or something. A lot of time when I'm talking to Ankit, we
would try to solve the problem right there itself.
[00:00:39] Nitin Singh: We have the data. Let's do it. Orlet's see the trend. OK, yeah, these cars are having a higher price. We need to
reduce that so you do all that analysis on the fly. It doesn't happen in big
companies. You say, we'll get back to you. Yeah, how it happens in a meeting in
a big company. You say okay, Tom, we'll get back to you in the next meeting.
[00:00:56] Nitin Singh: And the next meeting is after oneweek and the next meeting you would say, okay, [00:01:00]so we have achieved 50% of the result, but I think 50% is pending. And this is
the roadmap we have. So maybe next Wednesday, we can show you a demo. And the
demo, you say, okay, the demo is not 100%. It's like a still 80%. So that's how
it works.
[00:01:12] Nitin Singh: It takes time. There's a lot ofhierarchy so that's how it works. But in startup, it doesn't work like that,
man. You will have Andrew sitting besides you looking at the report, man,
right?
[00:01:22] Andrew Liew: So immediately you have instantand run the data immediately the next day. Pop up just like that. Is that how
it works?
[00:01:27] Andrew Liew: Oh, is that fast?
[00:01:28] Nitin Singh: I you what I think I think Mondaynight there was a scenario in pricing and all. Yeah. Whenever I joined, when I
came to office on Tuesday some strategy already changed, right? They were 24 by
seven, man, but they also, it's not that it's you work all the time, but you
also have in the office also a lot of time you'll have You talk, you go outside
and a lot of them are just chilling.
[00:01:51] Nitin Singh: We're talking to each other, justchilling. And
[00:01:53] Andrew Liew: I'm just curious because Iremember like Mark Zuckerberg used to say that when, Facebook started as a very
small company, he [00:02:00] always advocatefor very scrappy kind of approach. Just, do it quickly. You have an idea, just
do it, test it. But as the company gets bigger and bigger, it's almost like a
bank.
[00:02:08] Andrew Liew: Oh me wait, before you push outthis instance, before you. Commit this GitHub code, let's do some staging and
testing before this whole thing hang like where is that balance? How does this
organization grows to enable that dynamic changes? You know what I'm saying?
It's a
[00:02:23] Nitin Singh: sphere of risk.
[00:02:24] Nitin Singh: So I'll tell you what. Let's saylet's say you're a tennis player. Okay. You started playing tennis. Let's say
Alkaraz. Okay. He's a champion right now. But let's say he just, he's just
playing his first match. So he has nothing to lose. Okay. He'll play all those
shots, irrespective whether they're the Pacific Federer or or it's a Djokovic.
[00:02:41] Nitin Singh: He'll play all the shots he'll belike very carefree. But one, the same bag, Alkaraz has won 15 Wimbledon titles.
And that's it. And Roland Garros titles and all then he, would have a different
way of playing. So he'll have a lot on risk. When you, so that's what, when
you're a big company, you have a lot of things on risk because a lot of [00:03:00] people would ask you the question.
[00:03:00] Nitin Singh: There's a big hierarchy,everybody, the VP would call you, the SVP would call you, the head of world it
will call you up. Hey, what's happening? We need to get it done. That's not
there to an extent in startup because you're already, it's do or die. To start
with, once you start seeing the result, you try to create a process, right?
[00:03:19] Nitin Singh: And that is very important.Anything in life, or it or everywhere you need a process. Yeah, if you have a
process, if you have a schedule, if you have anything while raising a kid as
well, if you're raising a kid, if you have a process the whole day, how the day
would be managed, what's the schedule?
[00:03:32] Nitin Singh: You can manage kid as well, butif you don't have a schedule, the kid would wake up at would not sleep till
midnight and keep bugging you the whole night. That's important in your life as
a project as well. So you need to have a process where you would try to
streamline, standardize your assets.
[00:03:47] Nitin Singh: You'll have a data repository.You'll have a proper check in process. You have a proper KT process. If
somebody joins in, how I have, how would I be doing the KT knowledge
transition? So you need to have all these processes. And again, going back, the
[00:04:00] best place I've seen process beingimplemented is Naggaru.
[00:04:02] Nitin Singh: Oh
[00:04:03] Andrew Liew: yeah. Your career experience sofar.
[00:04:05] Nitin Singh: Wow. Anything, which is aboutmanaging people. I always credited to Naggaru to be honest.
[00:04:12] Andrew Liew: Okay, now let's talk about whatis your view on digital transformation and data science? What do you think 5
years, 10 years will look like? And based on, what do you think?
[00:04:21] Nitin Singh: See, right now, I think people,it would not be that long that a lot of work would be automated. And for
automation to happen is not that Chad GPT has come or the AI has improved a
lot. The reason is acceptance. Because of Chad GPT coming in. People started
realizing the positive of these machine learning models, not a negative
earlier.
[00:04:45] Nitin Singh: They were focusing on theaccuracy, the negative that in 5% cases it is wrong, right? So that was the
thing because of which what would happen is a lot of work which can be
automated. Where the intelligence is not that much required, they will be
automated unless [00:05:00] we have a true AI.A true AI would mean, see right now what GPT is doing, it has learned from the
data and is replying on the basis of that.
[00:05:07] Nitin Singh: Yes, I agree.
[00:05:08] Andrew Liew: It's it's an instance based kindof
[00:05:10] Nitin Singh: response. Yeah. And these kindsof models would not sustain in future. They're very heavy. And even I cannot. I
don't have time to train such a big model on my AWS account or GCP or even or
on Databricks, I cannot do that.
[00:05:25] Nitin Singh: So the next wave of model wouldbe a very lightweight model, maybe derived from these heavy models, something
which is transfer learning, something where you can actually improve fine
tuning of models. I think that would be the next wave of model coming, a very
lightweight model. When
[00:05:40] Andrew Liew: you say lightweight, you'retalking about in terms of the computing power, computing the resource intensity
of use, or you're talking about a specialization of use cases which, lightweight
version are you looking at,
[00:05:53] Nitin Singh: I tell you. Okay. So I thinkspecialization of use case is something because these models which are coming
in are very generic in nature. [00:06:00] Yes.And you need to customize it. You need to fine tune it. Okay, so you already
have a created model. Let's say you're trying to customize GPT weights on let's
financial data and all.
[00:06:10] Nitin Singh: Let's say you're trying to dothat. You need to fine tune it. Now the biggest problem with fine tuning is how
much fine tuning is good. How much we should do it or we should even do it or
not. So these are the questions which are not being answered yet. It's fine
tuning is not a straightforward task. You can do fine tuning multiple ways.
[00:06:24] Nitin Singh: You can freeze. All the layerscreate output layer or maybe unfreeze all the layers then train the model. I'll
tell you my experience. So I, in Dartner, I trained a speech to text model. Out
of the box speech to text model was good, but for a company specific data, that
model was not performing well.
[00:06:40] Nitin Singh: Because certain words which arenot in that vocabulary, the training data set, we don't model doesn't know
about that. So I find in the model when I find in the model, let's I have a lot
of luckily I had a lot of data. So I created a lot of data. But the more I
trained the model, the worst it's performance became because it was losing the
old, weights [00:07:00] for also changing whatit has learned.
[00:07:02] Nitin Singh: Now when I just trained the datafor 10 minutes to 20 minutes, it performance improved a little. So now this
thing can only only through empirical analysis behind it. So I think this is
still a problem. And even in chat GPT, what I feel is in these elements, it's
very difficult to control them.
[00:07:19] Nitin Singh: You cannot control them.
[00:07:20] Andrew Liew: There is a hallucination effect,right? Outcome supervisory error is a lot. What do you take
[00:07:25] Nitin Singh: on that? It will be there becausethese are weights. You don't control the weights. You can only create a output
layer, a guardrails to ensure GPT doesn't behave like that. Now that, that's a
feedback loop, right?
[00:07:38] Nitin Singh: The more you learn, the morebetter you'll get. Now Chad GP is like somebody, and I was talking to somebody
in one of the conference. So Chad GP is like a brilliant chap you have in your
company, okay? Which you don't want. Go and talk to client , you might say
something, wrong as well, right?
[00:07:53] Nitin Singh: So, we are still not at that AIlevel where everything will be automated. Yes. We are at a level where, [00:08:00] we understand the power of AI. Weunderstand the positives of AI. I, don't think they're going to replace any
human and all. People need to upscale. Okay. Even if there was no AI, somebody
else, something else would have come.
[00:08:11] Nitin Singh: You have to, upscale. You have tokeep learning. That's the only way to sustain, to create that differentiation
in the market. That's what I've been telling you since 2014, you do the work
where your work is differentiated. Okay. It's not, it cannot be replaced
easily. That's what you focus on.
[00:08:25] Andrew Liew: So to the audience out there, areyou trying to say that yes, people will say that I chat GPT seems to know
everything from law to medicine to coding. Now then it runs country to what you
say, right? You've got to keep learning and learn deep or learn wide. Is it
because that actually enabled like this, new language called prompt jet
engineering to speak the right context so that the.
[00:08:47] Andrew Liew: Error actually is small. Is thathow to explain a paradox?
[00:08:52] Nitin Singh: Yeah, so two things are there. Sofirst is the prompt engineering basically. Is we're helping model to understand
our question [00:09:00] better. Okay. And that'swhere the developer or the people who train the model are being very proactive.
They realize this is a good way to actually get the answer, which is fine.
[00:09:10] Nitin Singh: You're helping the model thatthis is my context and that's according to the model will give you the output.
So that's how the prompt engineering came and not. Only prompt engineering
came, there's a lot of other third party tools that came as well. The vector
databases, it's crazy man, when something new comes up, I don't know how the
whole industry just responds very quickly.
[00:09:28] Nitin Singh: Like in case of bit blockchain, alot of things came up. Yes. Here as well. So that's what's, happening, prompt
engineering. And that's the first point I was saying. Okay this, prompt
engineering is a way to get the right output, which is fine. I think we should
also help model to give a better output.
[00:09:45] Nitin Singh: We should not be very vague inanswering question. The second thing is intelligence. For example, I know Excel.
Okay. I asked at GPT. Okay. What do you mean machine learning? I'll give an
example of Excel. There's a thing of some ifs in Excel. Yes. I asked [00:10:00] what the how, do I use some if in Excelit'll give the right answer.
[00:10:03] Nitin Singh: Then I'll ask, how would I usemedian ifs in an Excel? It was the same answer. Okay, there's no median. It's
okay in Excel. Similarly when I'm asking the model about I need to create some
sort of tokenizer using transformer library and all in all. So I thought, ask
the question. Now, since the model is generalized, look at a lot of repetition
and then accordingly answer the question based upon those repetition, right?
[00:10:28] Nitin Singh: So that's what happens for a lotof them. What happens is model will give you the output based upon its previous
learning that you know, it actually works like this. But our life, the human
lives are filled with contradiction. It's like chemistry. A lot of outliers are
there which you cannot say that everything works in certain way.
[00:10:44] Nitin Singh: If X equal to Y equal to Z, thatdoesn't mean W would also equal to Z so it's, different. So, that's what but if
you know the field and you're using ChatGPT, you can use it effectively. If you
don't know the field, if somebody from finance, they cannot go to marketing and
do [00:11:00] a job well.
[00:11:00] Nitin Singh: So you still need to be good indomain, at least have a basic understanding. Then you will be able to use
ChatGPT very well. So you're compensating, so that's what I'm saying, you're
compensating for that much error in ChatGPT by your intelligence.
[00:11:12] Nitin Singh: So that's the two things we needto really understand while using this model.
[00:11:16] Andrew Liew: And so in that case, like youmentioned about that paradox is being solved because by increasing their
learning in a specific domain, I'm able to capitalize on chat GPT. Give me more
work, more output or more in that specialization.
[00:11:30] Andrew Liew: Exactly,
[00:11:31] Nitin Singh: exactly,
[00:11:32] Andrew Liew: Now coming to the nextinteresting question is like what considering now there's so many genre of AI
so wide spectrum, what do you think the future of AI will look like and which,
genre will actually like suddenly expand and which genre will start shrinking
or be superseded?
[00:11:47] Andrew Liew: Yeah,
[00:11:48] Nitin Singh: I think image analytics.
[00:11:49] Nitin Singh: Has already been ahead of anyother branch and with transformers, a lot of things change for speech as well
as for NLP. But I think image would still be [00:12:00]ahead of the two, types of data. So image analytics, see image analytics, we
started with convolution, CNNs, now right now we then have a transfer learning.
[00:12:08] Nitin Singh: And the reason C and the imageanalytics is much more faster because I still feel image is all about edges and
shapes. And neural, basic neural network can learn all those things very
quickly compared to complexity in NLP or language, which we have very complex.
Same holds with the speech as well.
[00:12:25] Nitin Singh: Now speech as well, people havedifferent dialects different way of talking, pronouncing, pronunciation. That's
a challenge there, but still speech analytics has also improved a lot. I think
we have to wait to a lot of new models coming from AWS and Microsoft as well.
So those are decent. They do very well on normal colloquial English, but
normally how you talk, but as soon as you put them to a test for a domain based
transcription, they're not good.
[00:12:52] Nitin Singh: Even in teams. If you go. If youtry to talk something which is very specific to your company they won't be able
to do that. But for English, the [00:13:00]transcription would be very good. The company called auto. ai, they are very
good in transcription. But again, when you go to a domain, it's very difficult
because those models are not trained that aggressively on that kind of data.
[00:13:12] Nitin Singh: But yeah, to answer yourquestion, I think image analytics, image based use cases would be ahead
compared to NLP or speech analytics cases.
[00:13:19] Andrew Liew: Like, computer vision, imageanalytics would be ahead. Is it because, like you said the, labeling or the
structure of classifying the data is already mature and tons of images because
of our mobile phones and storage devices making it?
[00:13:34] Andrew Liew: So much easy to explore the depthand the breadth in this AI research, whereas like transcription or speech or
NLP, natural language, is because the labeling part, the evolution of, let's
say Singapore, like they say, the work from home, like WFH is a Very new term
in the data dictionary and so just all these new languages have to be labeled
and structured in a corpus in order for this field of AI, what do you think
about multi model [00:14:00] artificialintelligence?
[00:14:00] Nitin Singh: That's what's happening. Adifferent type. See, transformers actually transform the whole game and
transformers, usage of transformer to handle multi modal data. And then coming
up with different architectures. Research is also very much respected nowadays,
mainly because of these things coming in.
[00:14:18] Nitin Singh: So I really give a lot of creditto things like that. Not because they brought something. It's about the kind
of, buffer they actually gave to the data science community, the researchers
that people would actually. Look at their research with respect. OK, this could
really do well. So that's the change which I really thank, Tadgp, to be honest,
how it revolutionized.
[00:14:36] Nitin Singh: Everybody knows about AI and allonce you see. So, that's what. So yeah I would say that this multi model they
are the, thing for the future because you need to simplify things. See, the
most solution to the most complex problem is actually always simple. And
Transformer is actually making that simple.
[00:14:52] Nitin Singh: And Transformer is Transformer isnot just one architecture. It basically, in the last 10 years, all the research
which has happened, like [00:15:00] embeddings,positional encoding, attention framework, sequence to sequence encoder decoder
format all those learning has been encapsulated in one model, and that model is
doing very well.
[00:15:10] Nitin Singh: But it's not easy to train thatmodel. You need really strong RK of the framework to actually train those
models. And that's where comes the problem because not anybody can train the
model. Anybody can train a CNN, a neural net based model. It's very difficult
to train those models. So that's why I say that in the future you will have a
lightweight model transfer learning fine tuning to be more used.
[00:15:34] Nitin Singh: Company would come up with theAPI, the Google would come with API. You will use those APIs to solve your
business problem. Fine tuning can also take place at a Google server, GCP
server, or maybe AWS server. They'll have the whole product in place. So that's
how I see. It would be in the future
[00:15:48] Andrew Liew: now interesting as you mentionedbecause the explosion of chat gpt suddenly enable All the big companies or
small companies everybody start to say I want to research ai I want to [00:16:00] build ai I want to apply ai then now whatwe are seeing Based on facts on economics.
[00:16:04] Andrew Liew: com or academia, right? Is thatthe number of phd researchers? Or the workforce field in the research in this
ai is shrinking is being poached to all these big corporates, all these mind
you splash. What do you think? Should we be worried? Because nobody's going
into learning the fundamentals.
[00:16:23] Andrew Liew: Everybody's looking at theapplication of AI. What do you think?
[00:16:25] Nitin Singh: See, I would say the reason notbe that what should be the word should I use? Not democratized, to be honest.
Now the research is taking place, but it's taking place with the business
objective in the mind. There's a guy called Andre Karpathy.
[00:16:40] Nitin Singh: Brilliant guy. I think he'sworking in Tesla right now. In the vision, he was one of the frontrunner. I
would actually look up to him and look up to his lectures, so people actually
are right. People are going to big companies, but they, those people are also
doing the research. But the only thing is that research is conditioned on a
business outcome.
[00:16:57] Nitin Singh: Yes, because ultimately whatmatters is [00:17:00] money, right? The bottomline, and that's the truth. That's the truth. Somehow I would still see it as a
positive because 20 years back when Mr. Hinton came up with the capsule network
or with the neural nets, maybe way before that, not many people actually put.
Too much mine on that one, right?
[00:17:16] Nitin Singh: He was ahead of his time, but hehad that architecture way back, right? But right now, that's not happening
right now. If something is good, would actually be implemented, right? Now the
people are being released with the code in GitHub as well, right? So things
have transformed. It has expedited the whole research process as well.
[00:17:31] Nitin Singh: But yeah, it's not, I would say,that generalized. But it's a good thing that we have a condition based research
where you're looking at a business objective as well. I think it's, fine. Yeah.
It's more efficient
[00:17:41] Andrew Liew: to be honest. I'm aligned withyou that it's more efficient. On the other hand, yes, having business outcome
means more practical impact that we're seeing in AI research.
[00:17:51] Andrew Liew: Of course, the challenge on theother side is there's always this danger. You look at Geoffrey Hinton, one of
the forefathers of AI. He suddenly left Google. He said [00:18:00] that if I don't leave Google, I'm not able to talkreally about the danger of AI being misused for weapon, for surveillance,
secret policing.
[00:18:09] Nitin Singh: What's your view on that? I'lltell you one very interesting thing. See whatever you have in the market right
now, all the technology. The actual level of technology is much higher than
that. What has been deployed in the market is something which you are testing.
You already have a three, four upper version in place, already in place.
[00:18:26] Nitin Singh: So don't confuse that GPD is thebest technology or DALI or new model, Claude 2 coming in there. They have the,
they're the best models. They are not. I always believe Microsoft is a good
selling. They know how to sell things and they have a good reach as well. The
Microsoft, everybody the good big market they have.
[00:18:45] Nitin Singh: So it's very easy for them tocross sell their AI products. My personal feeling or my personal belief that
Google is far ahead than any of the the company there is. And the kind of
technology which is already there. And you also in a way, validated that Jeff
is also saying that I [00:19:00] cannot talkabout the danger because it's hard to believe the kind of things we can do with
AI.
[00:19:04] Nitin Singh: Now, 20 years back, looking atobject detection, segmentation, extraction, text to image generation would be
like you're watching some AI movie. Those things are very much possible and
democratize. Anybody can go and do that. So that's what I'm saying. It's the
actual level of technology is much higher and there are risks.
[00:19:22] Nitin Singh: There needs a good governance aswell. The AI governance needs to be there. But for that, people need to come
together and come set up that those processes or those standards. But right
now, there's too much at stake for these companies to actually beat each other.
They would not go that way because they don't want to prohibit their technical
growth.
[00:19:43] Nitin Singh: You know what they're learning.So that's a challenge and it will be a challenge to be honest because you can
change face man The image you can what things you can trust 20 years back. You
cannot trust right now If a video is coming audio is coming trust that what to
trust them, right? So the truth is becoming murkier the day by [00:20:00] day, right?
[00:20:00] Nitin Singh: So that's the negative probably
[00:20:02] Andrew Liew: Just those I mean you and I werein singapore right now, but just to the audience out there in Singapore we just
passed a few legislation law about the online harm bill or the deepfakes bills.
And we even have this verification of identity because there's so many scams
going on, right?
[00:20:20] Andrew Liew: And any, every 10 people, nowthree people can get scammed, whether is it like, Oh, I'm your mom or I'm your
son. Can you wire some money to me? And then the guy look at the photo was look
so real. Like. How do you tell? Shouldn't we be concerned about? What is the
thing to take on
[00:20:34] Nitin Singh: there? See, technology can beuseful.
[00:20:36] Nitin Singh: We should we should work ontechnology in such a way that we focus on the right area, but we need to have a
guardrails to... Take care of the negative scenario. It's the same with the
guns. All the guns you're using, if you're using at a border fighting other
country because of some issue happening, it's fine.
[00:20:51] Nitin Singh: But if you're using that gun toshoot people or, school kids in a country, then it's not good, right? Yes. It's
all about how you want to use. Yeah, [00:21:00]and it's time. It's good thing that because of all the explosion taking place
due to Chad coming in other models coming in large language model, people would
start realizing the importance of having governance there.
[00:21:12] Nitin Singh: And if you don't have governanceit would be a very tough. Thing to manage the technology because like I said,
the truth actually is getting here. You don't know what's right or wrong You
see a video, earlier you would say, okay, this is what's happening You're what
you're seeing is the truth, but that's not the case right now, right?
[00:21:30] Nitin Singh: Technology, like I said, the deepfake is highly underrated because the deep fake which you have right now in the
market is not Which is actually the current status is it's much more advanced
Okay, just by basic logic, it's much more advanced and it is somehow not in the
hand of many people. So few people who know the technology who have done it
they have the models and all.
[00:21:50] Nitin Singh: Like I said you need governance.It's it would be very difficult in future, maybe after five years. How you can
regulate AI. It's, would be very difficult.