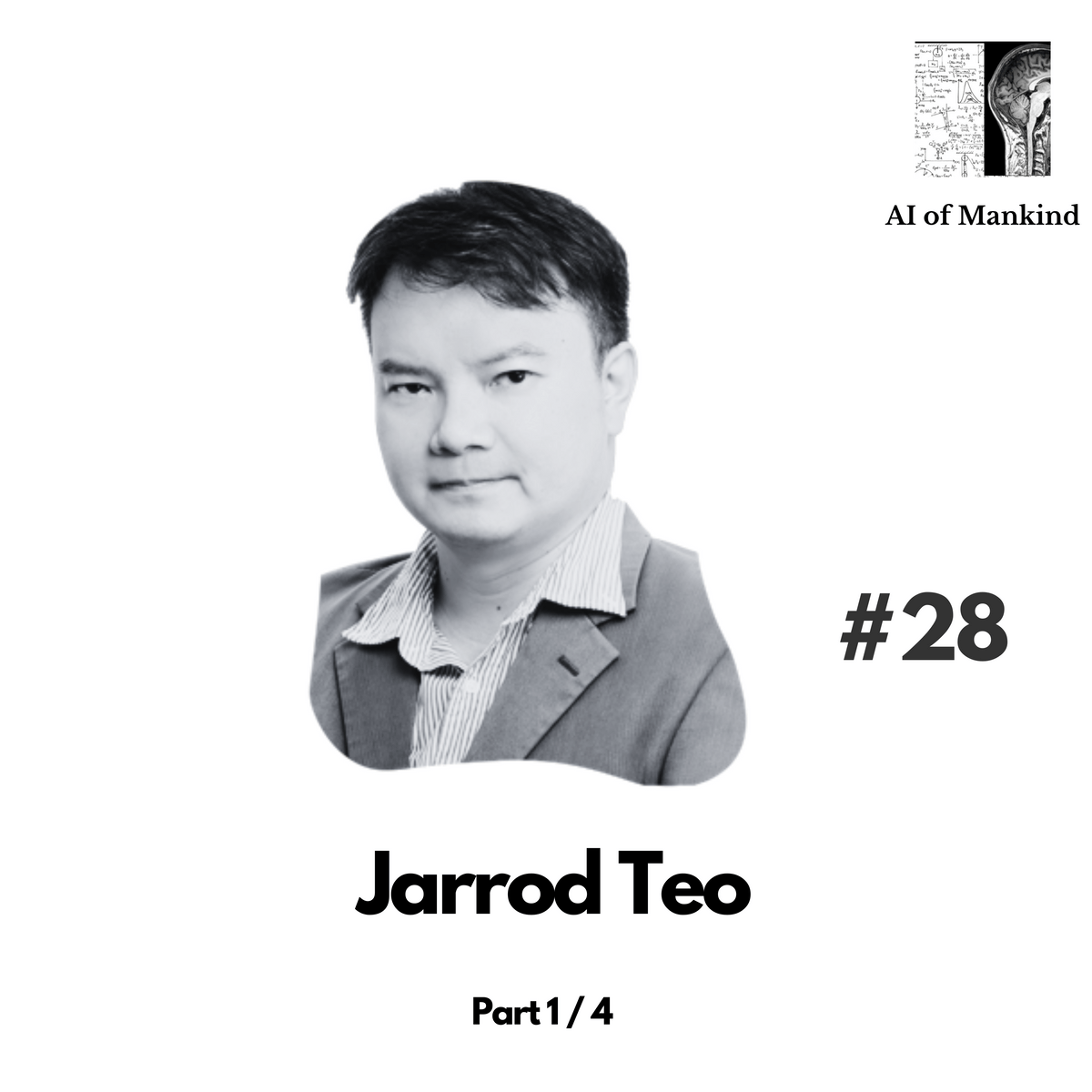
Trailer
Podcast with Jarrod Teo Part 1
Summary:
A social media post connected Jarrod and Andrew. Jarrod shared his career backstory on how he became the Alice in wonderland. Ok yes, how he eventually become the Chief Data Scientist from the day he decided to study statistics back in the 2000s when no one has a clue that a job like data scientist will have been created. He talked about how he went down the rabbit hole and eventually landed himself a role as the Chief Data Scientist at Direct Sourcing Solution. He also talks about an interesting project about using data to understand volunteerism in Singapore.
[00:00:00] Andrew Liew Weida: Hi, everyone. Welcome to the AI of mankind show where I share anything interesting about mankind. I'm your host for this season. My name is Andrew Liew. I work across four Continents and 12 international cities. Also, I work in tech startups across a range of roles from selling products, making customer happy, figuring out fundraising, making finance tick, building teams and developing sticky product. Apart from building startups. I've also worked in fortune 500 companies as a chief data scientist or technologist or people leader. You can call me Jack of all trades or master of learning. I hope to make this podcast show a great learning experience for us In each season, there is a series of interesting things where invite guests to share their views about their life and interests.
[00:01:09] Andrew Liew Weida: Now let the show begin.
[00:01:26] Jarrod: Very nice background .
[00:01:28] Andrew Liew Weida: We always enjoying time, right? Life is just too short.
[00:01:30] Jarrod: Sure,
[00:01:31] Andrew Liew Weida: okay. So yes, so thank you very much. Audience. Jarre is the guest today and he's actually currently the chief data scientist for dss, which stands for direct sourcing solution. it has about 17 years of experience on en coding statistics, data science and machine learning models. And IBM s spss, statistics and data trainer for three years has trained at least 3000 professionals. That's a big number. I'm not even close there. He trained multiple machine learning models with various Fortune 500 companies. We have some models running live and earning money for the companies for six years plus. Previously he has worked in Rakuten Nielsen Startup. Samsung,
[00:02:16] Jarrod: that's right. Yeah,
[00:02:18] Andrew Liew Weida: please. Thank you so much. As well, we've also mar about government agency and military science projects. Wow. This one's ,
[00:02:25] Jarrod: military science,
[00:02:25] Andrew Liew Weida: He personally setup three data science department from ground zero, which means starts from scratch and design 14 data science innovation that are making money. One of the innovation co causes or enabled a previous company to be valued at 13.4 million US dollars. Yes, that's right. Yeah. Oh. Before I begin asking you about this backs, ,
[00:02:51] Andrew Liew Weida: let me show you the audience, like how I even met you. I think we got connected on LinkedIn. That's right. LinkedIn. And I saw a post that you said something let me, show, you the, post
[00:03:02] Jarrod: so that we can it's about how to become a data scientist, right? Chief data scientist, not data scientist, but chief Data Scientist.
[00:03:08] Andrew Liew Weida: Yeah. Is it Okay let, me show you the, post. I think. Yeah, it was, I think it's about that. I think it is like, this is why you say you said, oh yeah, it's the Yes you and up going out of jobs. That's what a relative told me. Right here.
[00:03:20] Andrew Liew Weida: I'm
[00:03:20] Jarrod: getting . Yeah.
[00:03:23] Andrew Liew Weida: And you say that this is because professionals like doctors, lawyers, engineers, architects, accountants are portrayed as professional, driving big cars and living in big houses and local drama. These are great professions, but I wanna do something different. Yeah. So I end up as the second batch of Ians back in N us, which is stands for National University of Singapore. Long time. And you continue like to my great disappointment, searching jobs on jobs portal return, nothing do dot typing Statistics, gives you a screen and then you l Laugh out loud. I really [00:04:00] thought I will be out of jobs. I end up in credit score cut company and even went to trainer Opportunity eventually, which I thought it will be a training cashier, counter staff at Supermarket , but that was actually ibm, which stands for International Business Machiner S SPSS Training Opportunity. I still thought back then. This is just an honors job where no one will pursue, no one will like to look at the P value. Ah, no one will become a statistician or borderer to look at data fast many years later. Now you look at the, this year number is about 2022. My be statistics was the right choice. Amen. Yeah. Great. Of course to avoid going out jobs, I'm still learning every day so far across the last two years, I picked up four additional software. Of course, I will continue to learn and join this profession. And because of this post, it really caught my eye and not just me some of my podcast audience dropped me a LinkedIn and email and say, Hey, , this your guy is on your LinkedIn profile. Do you talk to him? He post this thing. That was seen one by one of the first graduate dudes, can you invite him to your podcast and get him to share the backstory because he said that he's doing a bachelor of and text. I think I want him to co university. Yeah. So Gerald, please share me like your backstory. How do you become a data scientist from the day you finished school when you finish, and us. Yes.
[00:05:35] Jarrod: Yeah. Thank you so much for inviting me to this podcast and thank you so much, listener for wanting to know more about how my journey is. So basically I end up with a statistics degree is because in junior college the statistics paper , that time my junior college teacher was actually walking around and asking Gerald, why you, why aren't you doing correction, right? So I was saying I got full marks . So, I, yeah, I got full marks. And then that's when I was thinking, can I do something with numbers to help business out there? Using numbers to actually tell, what is actually going wrong inside the business and then help them accordingly. And, that actually sparks me to go into statistics. And statistics is love and hate choice because the love is, it's. , is this mysterious, is this great? Everyone don't know What's that that time? Because it's especially I think somewhere around the AI winter somewhere around there, right? And then the, love is, mysterious. The hit is when you actually go on job auto, you actually eristics, it actually returns nothing.
[00:06:44] Jarrod: It's a very brave attempt to actually go and do this course when there is actually a local view that those professions that are successful are supposed to be doctor lawyers accountant, architect engineers. Even to today, some of the younger people actually still thinks that N US only offered this few choices of career so nothing about the profession. These professions are great. I almost went into law, actually, my English the time was actually quite good in JC. I did consider law but then I the GP or general paper, I'd actually score a b3 still. Okay. Not a plus. Yeah. So still maybe the entry is still able to go into law, but I was thinking I can't, my memory is bad, so I can't remember all the law , I can't remember the thick book of all the law things in Singapore. So, I was thinking, so what else can I actually do? And I was actually looking at back in school days, I will write one formula and then I'll actually derive the rest of the other formulas on a spot during exam. So I was thinking, okay, maybe mathematics. I don't need to actually do that much memory work. I just need to be logically sound. If I can make a coffee in the morning, then maybe I can actually do mathematics to see, take a coffee, put coffee inside hot water and add sugar. But if I can do that, then maybe I can actually be a statistician. So statistics is a very interesting job. And when I actually went into the credit score card company, it's not actually a local company it was actually an American that actually interviewed me. And then the owner is actually UK citizen. So, it is a very interesting setup. Then, the IBM one SBSS was actually a very interesting one. That is, The hit hunter didn't say much, so he just said train people. So the only thing that I can think of is actually okay, is to actually train on these AD and DC to actually count cash. So my as well, since I can't find any jobs, I go, trust me, I go around banging wars, a lot of peer hunters, and then they just say this is the first time I heard someone using numbers to help business. No, that's actually that, that those days and they say it's doesn't, seems to be possible. And then he, hunters was joking around say that no statistics to help business. I only heard it to help business if you don't know it, how we help business with numbers. It's, actually interesting that it get me to today when I actually take the courage to go IBM to actually take the job. IBM interview was very interesting. So because the credit score card company actually forced me to remember not just actually find quotes online. We don't have, I don't think we have Google back then so no, That time was YouTube . So, not, no sorry, YouTube, sorry, don't have Google, but is Yahoo. Sorry, not YouTube. Yahoo. And then people don't have active coding. Forums like these days. So, svss we, were actually, literally asked to remember the quotes. Yeah.
[00:09:50] Andrew Liew Weida: Rewind bit. You said that you decided to do statistics of mathematics because you, were aware that, or you think that your memory was so good. [00:10:00] Yes. So when, I'm just curious, so that moment when you joined the American Credit Score company and they say, Hey, Gerald, you gotta memorize this. How, was you responding? What were you thinking about that?
[00:10:10] Jarrod: My thinking is okay, that sounds bad, then I have to actually force myself to remember the code Frank, e f r e to repeat and repeat. I actually just really had to select e and all those things I could actually remember from scratch. And, thankfully for us business statistics, one thing is that if I can't remember the code, I just need to click, try and drop and then paste it. I have to command there, right? Oh, yeah. I hope this won't trigger some other software people to, suddenly just message me later. We feel that, I just feel that some of the software out there, if they can have click, try and drop and then paste the command in it, will actually help a lot of new data scientists. because then they will have no pressure on [00:11:00] remembering the code, right? DF something dot Yes. Value under score count, bracket reset underscore in index bracket. that is actually showing some sort like account value of that variable inside a data frame. If we can remove that and then allow them to actually look at the output and focus on it, it will help them to so understand what is Square? Understand what is doing independent test, how to use it. Lean in the question, logistic question. What is actually the key output that you need to understand what is host, I pronounce it properly, and adjuster ask and things like that. They need to understand those
[00:11:41] Andrew Liew Weida: I, totally align with you on this, is that if the, product or the that enable data science or machine learning is to be easy on the code, low code or no code, drag and drop, then to really focus on memory, then people are more interested in the math, the intuition behind the code, right?
[00:12:02] Jarrod: Yes, that's right. Yeah.
[00:12:04] Andrew Liew Weida: And, come back. Coming back. If I remember correctly correct me if I'm wrong, it's like I remember S SPSS is also one of those new, drag and drop or new click here and click there. Cross away.
[00:12:14] Jarrod: Yeah. So why that time they wanted you to memorize stuff? Because you see initially I was thinking why do why, did that UK boss wants me to remember the code is because then I re I slowly realized that if I click try and drop, I can actually get, for example, open file. Yes. Is just click and drop. But then if I actually do the coding, I can get file and then that file tree and then the file name, I can do a sub command slash keep certain variable slash rename or slash drop. This is, this sum of the commands allows you to do something additional, which the click and drop actually don't allow you to do. So it opens up the software to become even more powerful, do to repeat, do repeat and repeat is actually some sort like looping code which allows you to actually pair wise two command calculation for two set of variable. So two, two sets of variable V1 to V 10 and then H one to H 10. You wanna actually do some compute pair wise? Yes. V1 assigned to H one or V2 assigned to H two, then do repeat and repeat actually helps a lot, but then this is something which the clicker and drop, as far as I can remember, it doesn't actually had that functionality.
[00:13:30] Andrew Liew Weida: So it's almost am I say it's almost like they don't have those everybody, most people use Microsoft Excel, right? Those Microsoft Excel. You have this macro that you, after you click a few things, then it records the visual basic script on the backend. Yeah. Then because just update, so they don't have that sort of macro recording click, and then adjust the code, understand that and back.
[00:13:50] Jarrod: So basically what happened in Air SPSS itself, statistics, airspaces statistics is allows that kind of setup of remembering code and that's where picking up other codes like the coding modeler the coding in arteries, even a coding in Python it's actually easier to actually remember. I mean it, it's second to nature because I've been typing this for 17 years and then to actually learn new software command it is actually becoming much more easier. It is an IT manager back then in IBM SS did actually told me this. You learn one quote, you learn many code. This, you just need to actually take care of the grammar. So he is actually quite right about that. .
[00:14:31] Andrew Liew Weida: Is it because syntax or the structure of coding? I think almost once English, Spain I mean it's easy to pick Is it something like.
[00:14:40] Jarrod: Something like that. So, basically what happened is that let's actually say do AIF is actually in S spss, but then if AIF is actually in Python, yeah. It's, it serves as a same functionality. It's based on a certain condition. You execute a certain action, right? So, then because of that, I learned in s it's easier for me to actually pick it up in Biden and, things like that. And I was actually using SA as well r long time ago. But I don't claim expert. It sa in r i just actually just stick with SS for many years. Why I stick with one software is because I am focusing a lot on picking up how to solve business problem in a very unique manner. So there are a lot of experts out there and, their focus is actually not on tools. Their focus is how to solve business objective. And that focus alone is very powerful because they share a lot of very unique thoughts about that. And this is actually what. , I can say that based on the linking post that we see daily, most of them are just actually looking at, which software is better? How do we execute this code? Yes. You see it, it actually takes away the very important thing, which is how to solve the business objective. I, don't see much of this discussion on LinkedIn. I'm not sure about you, but I don't see about, so we have this problem and then this is actually the unique way that we solve it. That is we actually collect survey and then we do a model of it, and then we scroll back to the entire database, something like that. We don't actually see the accounting. I think
[00:16:14] Andrew Liew Weida: I'm aligned with you on the general view that I got the sense that there's two schools out there. One school is the majority, one that we always see on LinkedIn. Anybody that's a data scientist will write all this, for example, this custom algorithm or, and they'll spell the they use Python. They'll put out the Python code or article code, and it's always along the, what does this algo do? But they don't yeah. But the other score thought is they will look at, okay, what's the, like you said, what's the business problem? Is this solving problem that increased revenue, reduced cost, reduce risk, and then you'll backtrack to whatever that function is. And then picking out, like you say, picking out that specific statistical technique to deploy. and visualize it. Then there's two score though, right? One is the, I'm just focusing on the algorithm focus. The other is I'm focusing on the business focus, right? Yeah. And then picking out the tools that you are familiar with because you are, like, for example, you explaining s because it allows you to focus more on the business and then picking out the right specific subset of solution to the business problem.
[00:17:22] Jarrod: That's right. Yeah.
[00:17:23] Andrew Liew Weida: So what is the, interesting thing that when you pick that review throughout that career, in that tree business setup that you did for different companies and for clients how does that help You And how does it help the client in a sense?
[00:17:39] Jarrod: Setting up department is a different monster together, . So, basically what I can say is that data scientists actually might go through a few phase. I'm, I will want to say software and tools are important. So this is actually where you start off first. You, learn the, software inside out. You learn how the tech stack actually works. This is fine, right? Then this is actually a journey to become some sort, like a data analyst and you actually do some simple report and hopefully survey as well, because As you progress into a data scientists, you don't know, have to write a proper survey, or questionnaire, then it's very funny. But then of course it also depends on what kind of data scientists you are. Because when you actually move on to data science, as I actually had tell people a lot of time, that is there are five schools of data science. We might eventually classify it like that. We might Okay. Depend on the industry. So numeric science, there's tech science, there's image science, there's video science, and there's vocal science. There's only one person that I've seen so far that has actually deployed practical usage of all these five data science, which is Andrew Ung if you remember the big, green, big China professor, right?
[00:18:51] Jarrod: Is that what Yes. He, actually sent up the show two or something like that. He's actually a robot that represent the company to win competition against the contestants who are super genius. So he actually deploy numeric science, tech science, video science focus science and image science inside the robot. yes, it's actually his robot is actually doing very well. So, then as data analyst to actually proceed to become a data scientist, then you have to actually think, oh, you can actually become a hybrid. You can become someone who specialize in numeric and. or text and video, it depends, right? But ultimately you have to actually think of a usage for them. So then you will slowly also think about, so what domain you want to, what industry you want to actually focus on. You can't be focused on everything. You just actually pick something that you like. That is, you might actually be someone who is into banking or maybe insurance or mean military science. I, do military science. Okay. There are actually a few industry that I focus on too. I focus on retail e-commerce. Okay. Banking, insurance, mobile. Yeah, mobile phone. Yeah. Another one is telco and military science. So around like seven plus. Yeah. So seven years later. So my focus is always b2c. And then I will always look at big data to actually look at it. So then, when you journey into a data scientist, you will actually start to actually know how does the tech stack function altogether, right? Because you have collaborate with the different IT departments inside the different scope of thoughts, cloud engineer data engineer or, things like that. So, you have actually done that. Then you have to actually ask yourself, do you want to actually go front end or you want to actually remain back end, right?
[00:20:35] Andrew Liew Weida: You mean the data science front end data science backend. What does that mean?
[00:20:37] Jarrod: Yeah, because this, so backend data scientist is someone who actually called nick models and then and then do analysis. But then a front end data scientist actually is different. It's, he's someone who will represent the data science department to present to investors network and maybe say even to sell to businessman at 10 business meeting. Understand from businessman point of view what is actually a objective that want to solve. And maybe say if he can on the spot create blueprint that is, oh, this is rough blueprint that we we might actually offer to actually solve your business problem. So, for example I, had actually met with cans last time. He say, I wanna grow my vegetable well, right? So this is actually not a statistical question. I want to grow a vegetable. Is not a statistical question. So I ask him, I say that, okay, I'm gonna grow your vegetable. Fine. Can I know how many methods you have to grow a vegetable? He say, three methods, right? That ask him, can I know if you have aging days, years, the kind of thing. Yeah, I have aging days. then I was, I told him on the spot. I said that so these three methods are independent of each other? Yeah, independent of each other. Okay, fine. I was telling him an over H O v homogeneity, of variance, and then we'll actually test that whether is significant or not. If then we actually move onto overall average, then we actually look at post hoc, then maybe say, look at LSD or games hoel, but depends on the different situation of the post. He was saying, Jerry, what are you talking about? He's a chemist. So I was like this is statistics . I said, don't worry, give me your data. I will teach you in the class itself. So that was actually I, IBM m s
[00:22:19] Andrew Liew Weida: vs. Okay. Now, I get a clear understanding of what you mean by front end and backend data science.
[00:22:24] Andrew Liew Weida: Yes. Okay. Cause I think in, my world I, see as differently I, see as those that like, oh, you are backend is very technical data scientists. And those on the front, I call them business savvy or client-friendly data science. Because client-friendly, they need to speak to the business, but they need to translate the two languages. They is business language, translate back to data science, then translate forward back to the data the business guys. To the business guys. It's almost like a data business translator. And then the backend guys there the, technical data scientists are more okay. this client friendly data. Talk to me in the dataset link, say, what are the different methods? Is this a, computer vision problem? Is this a, like you say, a tax problem? Is this a linear regression problem? Yeah. So then, you'll think of, okay, maybe why is where I need to then he communicate back, oh, can I do this method or cannot do this method? Then the, business friendly desk need to go back to, like you say, the farmer. I think we can use camera to solve your problem. Yeah. Or, I think we can use, if you gimme water and this internet device, we can solve this problem. Yeah. So yeah, something like that. Continue. You are train and in s spss and then instead how do you go from training?
[00:23:44] Andrew Liew Weida: What's after that? What's after, training s sp
[00:23:46] Jarrod: statistics training, because I happen to train a bunch of professionals and then I happen to train the owner. So the, then the owner say, would you like to come to my company to to do [00:24:00] data analytics? I say, why not? Because I met up with a client who actually told me this. He said that in general, now you are a trainer. If Dennis is later, you're still a trainer, then you are failure . Why?
[00:24:11] Andrew Liew Weida: Why does he say that?
[00:24:12] Jarrod: Yeah. I was asking him why. Then he say what you train now is actually a. Won't you want to see your practical usage be used? Do you want to try your theory in practical usage? That'll be more fun, right? Then say, that's actually true, eh? So now I'm just training people on how to actually execute. Why not? I actually go to the front and the business problem site to actually try to execute what I think that I train people is supposed to be useful. That's actually very amazing part with, I step off Svss and I go on to join my client company. And then that client company was actually very interesting when I was actually on that in the interview that day, I was actually interviewing for junior role. So he say, Jarre, if you join today, I will promote you now I'll double your pay
[00:25:00] Andrew Liew Weida: Alright. He say that. Okay. First is, it a in-house role ?
[00:25:03] Jarrod: In-house role. So if you join today, yeah, if you join today I'll, in, I'll promote you now in in promote you now and I'll w p say, okay, why not ? So I just joined you see, promote you now on the spot.
[00:25:14] Andrew Liew Weida: And then
[00:25:15] Andrew Liew Weida: w your interview?
[00:25:16] Jarrod: Yes, during interview.
[00:25:17] Andrew Liew Weida: So actually in, in, did it happen?
[00:25:20] Jarrod: It happened . So then I joined them and then I joined them and I was leading a team of Slightly a junior statistician. I was actually teaching them coding in the company. And I was actually teaching them coding s spss. And then I was actually teaching them statistics notice because during the time, yes, it is still says, and s spss Python and R is still, is there button is not as user, it's not actually so widely used now as of now.
[00:25:45] Andrew Liew Weida: And, but coming back to that, time the, client that brings you on board is it a a military defense science company or is it some Fortune 500 companies are all kind of companies? They
[00:25:54] Jarrod: No it's just research house. Oh, research house, okay. Yeah. So this is actually something which a site advice to, some young data scientists as well. Yes. To not actually go straight to become a data scientist, be a data analyst first because this allows you to have that. That area for you to, that, that small space for you to, learn quietly. Because once you become a data scientist, everyone starts to look for you , and you cannot learn in peace. You might actually end out in pieces ,
[00:26:26] Andrew Liew Weida: We, come from a different perspective and different lenient dots. Yeah. So what's the difference between a data analyst and a data scientist?
[00:26:33] Jarrod: So data analyst to me is actually someone who is actually managing the pipeline. Like he, he must know how to execute the extract the data, clean the data and, is some sort like the starting of the pipeline ETL basically. So then know how to actually transform it and then into clean data and then load it into a report, right? Then present as a storytelling manner to actually executive to tell them what is actually the insights of the data itself. Then this is actually where the data analyst, if he actually has passed this, he will actually start look at things like feature engineering, feature selection, and then they will actually look at, not because statistics is like that, , there's univer, there's five variant and there's multi variant, right? So they were actually starting not to just look at univer is one variable, but five vera is two variable, which is two business factors. Multi vera is look at multiple factors together, business factors. So then you will journey from the transition of end analyst to data scientists. You look at multiple business factors together.
[00:27:38] Andrew Liew Weida: Ok. So just saying that the difference between a data analyst and a data scientist is one is looking at single factor and one is looking at multiple factors by, very and reporting. Ah, okay. And then data scientist is someone who is able to deploy machine and model order. This is just a personal opinion.
[00:27:56] Andrew Liew Weida: Yeah. I know there, there could be actually some opinions out there of companies that actually require a junior person to actually straight on to become a junior data scientist and start from there, deploy models in others. But I just feel that it's, actually less stressful if you start to look at uni and bi.
[00:28:15] Andrew Liew Weida: I, think I agree with you on that part. That is less stressful to look at uni variant or by variant. But I think the difference is that my view is I'm also different is my past experience and my current experience. Yeah. Is data analyst is looking at very small data sets. Yeah. And doing very simple what is happening data description, data visualization, reporting. And, anything is more complex than that. That means that anything that is asked about what will happen to the future, what if I change this, what if I change that, how it look like. That's right. Those like predictive modeling, those that to test causality, those that the classic everybody like use this a b testing type. Those I think is more like a data scientist because he needs to think of different algorithm, test it, and even using huge data sets.
[00:29:05] Jarrod: Yeah. So this is correct because you see why we start with small data as well. It's because for someone who have no experience in data, at least when, if a small data, you run a command, if a live button in all those, you can easily see how the command actually works out, right? Yes. You can easily see as well that is how does actually the number actually works out on some simple statistics like high square two pen test and Nova and things like that, or manova and cova and things like that. But then if you jump straight into big, data, then good luck to you. Number one is you actually gain attention to everyone in the company. Oh, he's a data scientist. And then everyone start to actually look for you. , you won't have time to self-taught and say why is it like that? Why is my coreman actually not behaving well? Is my common having some or [00:30:00] I always. if the commercial show error is actually a blessing, , if the command don't show error, then that's it, cause it runs into logical error. You don't pick it up. Then that's it for your machine learning model.
[00:30:11] Andrew Liew Weida: I, like the way that you think about it. I think it's always better to learn from failure. Failure. You teach us about success, right? Yeah. Cause you wanna know what's going on, right? Yeah. Now coming back. So you so the role that they, they bring you on was from data analyst immediately promote you to be a, senior data scientist, senior data analyst. It was a research. What's the generalized business topic that you guys were solving or researching for the client or the end user?
[00:30:37] Jarrod: Yeah, basically it's related to government sector. So it's always about collecting survey and trying to actually understand about what the respondents feel about the events that the government actually set out. I was actually involved in PGO to NI one project. and Poko one project was supposed to be under H db and yes, hdb and I went to HDB to present to the ceo o. So interestingly that project was actually presenting to director. It goes to managing director, it goes to finally the CEO e o, and then the c e o decide to say, okay, let's actually bring it to the steering committee, which means it involve various government sectors to actually, which is n ea and other sectors to actually just align with what the respondents want on the PO Golden one project. What are they looking for in the campaigns and, things like that. So you can imagine the impact of a data analyst is not just actually something that you think that is small. It can actually go all the way up until like that. Another project that I was involved in I if I can remember only, I'm not the direct person who is, who started entire thing, but after the project was near end I, took over a bit and, I was cleaning up things a bit and I was actually the one that my boss actually say, Hey, look, I think I need you to present to prime Minister Lisa. So . he said that if his flight is late and then no one goes, then I will be the one going then say, okay, fine, I'll go. Then he asked me that time do you want any analyst to follow you? I said, no, it's fine. I'll go alone. . But, and yeah, the directors actually on, that one is People's association. So there are directors that actually present to leasing loan. So we just pass the report. So you'll be, you'll require a fun experience to present to Prime Minister Lee.
[00:32:37] Andrew Liew Weida: Like, high profile people. How, does it feel? Is this is, that the first time that you present to a C-suite guy are high profile stakeholders? Yeah.
[00:32:47] Jarrod: No, Because I, I did train people from C-suite last time in the IBMs business. Ah. So, I'm so and so like numb, do it really . I see them every day or maybe they're directors or managing director every day. So, when I was training a managing director last time in, the certain company, I was showing her position three. say if this person is a female and then she earns this much, she's actually likely to be a high spender. Things like that, right? Yes. So she said, Jared, I can actually use multiple cross step to come up with the same conclusion as you, right? I said no, Without my decision tree, you won't be able to know that. This is the profile number one. Number two is, if you have 2000 over factors, good luck to you to use the Biva permutation to come up with the same thing as me.
[00:33:42] Andrew Liew Weida: Factors that he must need a lot of data set, right? A lot of trial and error of, oh, it should be gender we spending gender, we wear stay and north.
[00:33:53] Jarrod: So east west. And and then after that, when she heard that is, oh, I, the 2000 business [00:34:00] factors, then I have to actually come up with a different permutation. Then I can come up with the same division three profile as Jared. She say, okay, say no more. Then she say, what? No, tomorrow I'm gonna actually bring my entire team here. So I actually received two teams of people coming to be trained under me. . Yeah, interesting, huh? That means you have very sharp students yeah. That trust you. Yeah. Past your age of your knowledge, right? Oh this is skillful. This is a skew That is interesting. That is because I'm being tested consistently in class, right? Yes. I was actually being have I, I train up this very sharp thinking whereby I can like on the spot actually come up with response to the person like that. So on the spot I can actually tell you, oh, it should, be not be done this way, it should be done this way. Maybe things like that will be better and do some recommendation. That, so I do encourage young statistician or data scientists to try some training. Not too difficult one. Yes, if you want to assist those newies, why not? But then make, just make sure that you are training right thing. Don't the guy that the blind guide the blind, then you are training wrong things. And then they were actually causing me. What was
[00:35:20] Andrew Liew Weida: that class of, advanced students or It was like introductory class. And you met with very sharp student already. Oh, that was actually advanced class actually. Ah, okay. That was, sorry, experience. But it's definitely true. The
[00:35:34] Jarrod: first question. So then, another thing is that I did actually manage to train to to, present, not to prime Minister Lee, but present to a lead family member. So I did actually present a statistical model to Lee family person. And then that one was actually helping the company to actually find out why volunteers don't want to actually volunteer at all. How do we attract more volunteers?
[00:35:58] Andrew Liew: Hi guys. Thanks for listening to part 1 with Jarrod . In Part 2 of the episode, Jarrod shares his digital and data transformation case on helping the government with volunteers’ acquisition strategy for non-profit organizations. He also shares a project that makes money at the expense of others’ privacy. At the same time, he shared a successful data transformation story of how a digital marketing campaign is done in a country of unrest. if this is the first time you are tuning in remember to subscribe to this show. If you have subscribed to this show and love this. Please share it with your friends, family, and acquaintances. See you later and see you soon.